When Algorithms Wander: The Impact of AI Model Drift on Customer Experience
One of the challenges with AI models today is that once you release the model into the wild, it can “drift” and become less effective. Researchers from UC Berkeley and Stanford recently released a study that revealed the performance of advanced large language models (LLMs) has experienced a dip, raising questions about their reliability and stability.
This challenge emerges just as the significance of conversational AI capabilities is growing exponentially and receiving greater investment as organizations look to incorporate the technology as part of a long-term customer service strategy to reduce reliance on live agents. As these organizations seek to harness the power of conversational AI, they must navigate the risk of AI models gradually losing their effectiveness. This raises a critical concern – what happens when a conversational AI system, meant to enhance the customer experience (CX), starts producing subpar interactions due to drift?
In this article, we’ll explore the risks and dangers of model drift on CX and how organizations can navigate the balance between leveraging AI advancements and maintaining exceptional CX standards.
Model Drift: The Silent Saboteur
In short, model drift refers to the gradual degradation of the performance of an AI model over time. This can be caused by various factors such as shifts in user behavior, evolving patterns in the input data or changes to the environment the model operates in. As the AI model encounters different and unanticipated information beyond its initial training scope, it can–and eventually will–encounter difficulties in maintaining its accuracy and efficacy. For example, a speech recognition model trained on specific regional data may struggle with accents or dialects not present in its training set.
The Impact of Model Drift on Customer Interactions
Model drift poses a considerable risk for organizations relying on conversational AI or chatbots for customer interactions because they could start producing responses that are irrelevant or inaccurate. This not only jeopardizes the CX but also raises questions about the reliability of the entire system. If an e-commerce chatbot suddenly encounters a surge in complex technical questions due to a product launch and it hasn’t been trained or tested for these new patterns, it may struggle to provide accurate and helpful responses. This will likely result in customer frustration and potential business loss.
Navigating the delicate balance between leveraging AI advancements and maintaining exceptional CX standards is top-of-mind for many organizations today. On one hand, AI-powered chatbots offer unprecedented capabilities to understand and respond to customer needs. On the other hand, overlooking the potential pitfalls, such as drift, can lead to a decline in customer satisfaction and impact the bottom line.
Steering Clear of the Dangers of Model Drift
Organizations can mitigate the risks of model drift by adopting an automated, continuous testing approach. This involves regularly testing the model to identify and address any issues before they occur and helps organizations detect early signs of model drift and prevent potential disruptions in performance.
A key aspect of this approach involves evaluating the model’s ability to understand user intent, which refers to the specific goal or outcome a user intends to achieve when they engage with AI. Understanding intent is especially important in customer service scenarios. Unlike live agents, who can easily comprehend a customer’s intent, AI chatbots can face difficulties due to the nuanced and diverse ways in which humans express themselves. Ensuring that a chatbot can consistently interpret intent requires ongoing training and fine-tuning. By regularly training the chatbot on a broad spectrum of potential user interactions, organizations can create a more resilient bot that is better equipped to handle diverse scenarios, user preferences and the evolving intricacies of human communication.
Organizations should also implement sophisticated natural language processing (NLP) techniques. NLP enables AI systems to comprehend not only the explicit words used but also the underlying context, emotions and subtleties that shape human conversations. NLP plays a key role in deciphering the intent behind customer queries. Whether a customer is seeking information, expressing a concern or making a request, NLP algorithms can analyze the language used and discern the underlying purpose, allowing the chatbot to generate more contextually relevant and helpful responses.
Confronting Model Drift for Lasting AI Reliability
The risks of model drift pose a significant challenge to the long-term reliability and effectiveness of AI-driven chabots which can ultimately have a negative impact on an organization’s bottom line. Forrester research shows that after just one bad bot experience, 30% of customers said they’re more likely to use or buy from a different brand, abandon their purchase or let their family and friends know about the poor experience they had. By proactively addressing model drift, organizations can ensure that their AI models continuously deliver reliable and accurate results, maintaining the integrity of their AI-powered bots and thus, maximizing their ROI from AI.
About the Author
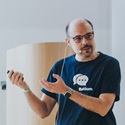
Christoph Börner is a multi-organizational founder, developer, tester, speaker, and in his
spare time, a pretty great drummer. He is the Senior Director of Digital for Cyara and the co-founder of Botium, the leading industry standard in test automation for chatbots, voice assistants and conversational AI.
Sign up for the free insideBIGDATA newsletter.