Leveraging AI to Design Fair and Equitable EV Charging Grids
By: Ankur Gupta & Swagata Ashwani
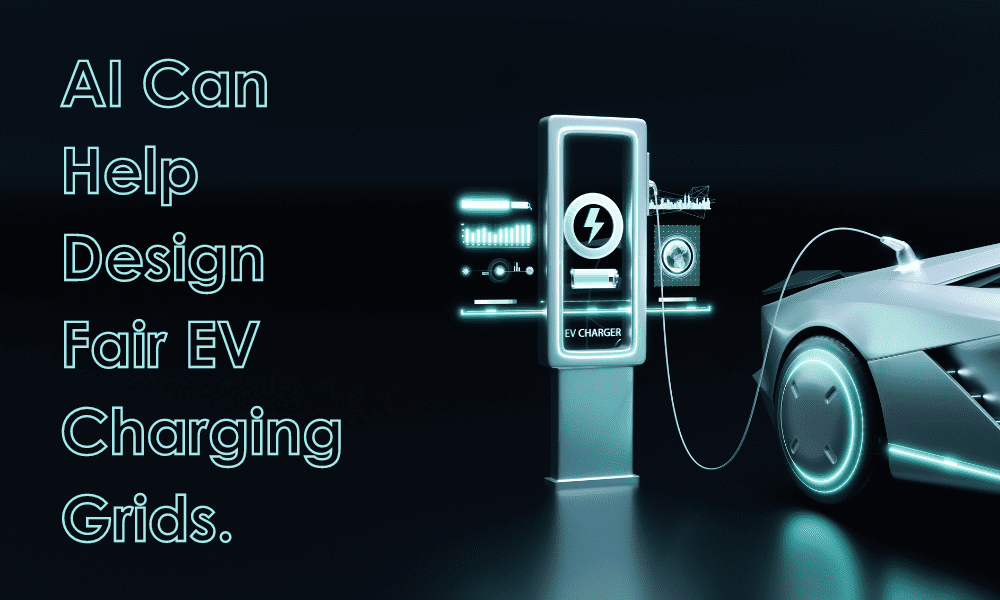
Image by Editor
Artificial intelligence holds immense promise for revolutionizing the accessibility and availability of electric vehicle charging. The demand for EV charging is exploding as the transportation industry undergoes a massive shift towards electric vehicles. Over 6.5 million EVs were sold worldwide in 2021, accounting for 9% of passenger car sales. That number should exceed 25% by 2030. A recent analysis estimated that the number of charging stations required to to satisfy the charging demand would need to grow 10x by 2040 [1].
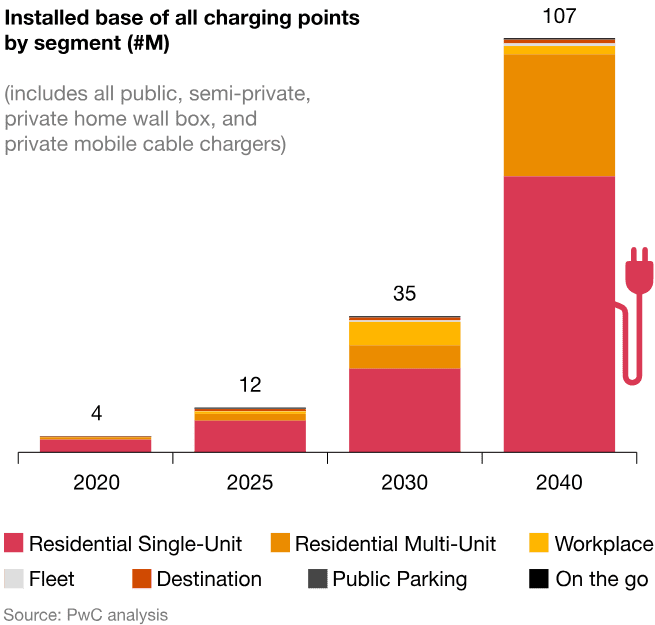
Figure 1: Projected demand for EV charging stations by type
AI algorithms can help create a smarter, more responsive charging infrastructure. However, as we welcome the benefits, we must also navigate the rapid deployment, we also need to ensure that it aligns with values such as fairness, transparency, and accountability.
The datasets that feed into AI models would base their recommendations on current EV adoption in those areas, EV demand and expected charger utilization. However, we need to control for bias based on socio-economic factors to ensure that new stations that are placed on the grid enable fair and equitable access.
There are also myriad scientific studies [2,3] that discuss how AI and machine learning can be used to help planners decide where to locate EV chargers and what type of chargers to install. Designing an EV charging grid is a complex problem and various factors are at play including
charger location, pricing, type of charging standard, charging speed, energy grid balancing as well as predicting the demand. Let’s dive deeper into the key aspects where AI can help guide us in making a better decision.
1. Optimal Charging Station Placement
AI excels in processing vast datasets and extracting meaningful insights. This capability becomes particularly valuable when determining the optimal locations for charging stations. By analyzing factors such as traffic patterns, population density, and geographic data, AI algorithms can strategically place charging stations to maximize accessibility and user convenience.
For example, EV charging stations might be needed along busy commuting routes, near major highways, or in areas with high concentrations of EVs. High-density residential and commercial areas are likely to have a higher demand for EV charging stations. AI can analyze demographic data and population density maps to pinpoint these areas. For the analysis, the datasets need to incorporate future trends in EV sales, population growth and urban development.
The Best Site for Charging Stations:
AI algorithms are superb at analyzing big data. They can help to determine the best areas for EV charging stations. Various aspects are considered in this assessment including:
- Traffic Patterns: AI looks at traffic flows and congestion levels so as to identify areas with high usage.
- Population Density: The priority is given to places with high population densities thus ensuring that there is maximum accessibility.
- Geographic Data: This involves examining the physical terrain and constraints of urban planning to judge their appropriateness.
- Existing Charging Station Locations: In order not to saturate any area and maintain an even spread.
- Predictive Analysis for Future Expansion: AI uses trends in electric vehicle sales, demographic shifts and urban development to project future requirements that guide long-term planning.

Figure 2: Heat map showcasing distribution of EV charging station in the US
2. Demand Prediction
An effective demand prediction strategy is crucial for optimizing the placement and operation of charging stations and is essential for several critical reasons. Firstly, accurate demand prediction allows for the strategic placement of charging stations. By forecasting when and where charging needs will be highest, AI-driven systems can optimize the geographic distribution of charging infrastructure. This ensures that charging stations are conveniently located in areas with anticipated high demand, promoting accessibility for a diverse range of users across urban and rural landscapes.
Secondly, demand prediction contributes to effective capacity planning. By analyzing historical data and incorporating factors such as seasonal variations, time-of-day patterns, and user behaviors, AI can help determine the optimal capacity for each charging station. This ensures that the infrastructure is designed to meet demand without causing overloads or inefficiencies in the power grid. Listed below are factors that feed into demand prediction.
- EV Charging Transaction Data:
- Details about each charging session (time, duration, location)
- Energy consumed per charging session
- Type of charging (fast charging, slow charging)
- Traffic and Mobility Data:
- GPS data from vehicles to understand travel patterns
- Traffic flow data in different areas and at different times of the day
- User Demographics:
- Age, gender, and residential location of EV users
- Weather:
- Weather conditions can affect driving patterns
- Socioeconomic Data:
- Income levels
- Urban versus rural areas
Predicting demand is crucial for user satisfaction. Users benefit from a charging infrastructure that aligns with their needs, minimizing wait times and providing a seamless experience. AI’s ability to analyze diverse datasets, including user behavior and preferences, allows for personalized and user-centric demand prediction, enhancing the overall satisfaction of EV owners
3. Dynamic Charging Pricing Models
Traditional fixed pricing models may not harness the full potential of a dynamic and responsive charging grid. AI can analyze real-time data, including energy demand, grid load, and user behavior, to implement dynamic pricing models. This not only optimizes the utilization of the charging infrastructure but also encourages users to charge during off-peak hours, promoting a more balanced and sustainable energy distribution. A research study [4] on dynamic pricing scheme based on the Stackelberg game for EV charging stations led to the conclusion that a well crafted pricing scheme can lead to reduction in the selling price of charging station while increasing the station’s profit; a win-win for both the consumer and the provider.
Components that feed into a pricing model:
- Energy Demand and Grid Load: AI algorithms can utilize real-time electricity demand and grid load data. During high demand, prices can be increased, and vice versa.
- User Behavior and Patterns: Analysis of historical charging data, including frequency, duration, and preferred times for charging, helps predict future behavior and adjust prices accordingly.
- Time of Day/Week and Seasonality: Prices can vary based on the time of day, day of the week, or season, considering typical usage patterns during these periods.
- Type of Charging (Fast vs. Slow Charging): Different rates can be set for different types of charging.

Figure 4: Pricing for EV charging stations in the US
Dynamic pricing models play a role in affordability and accessibility. By offering lower prices during off-peak hours or when renewable energy sources are abundant, AI-driven systems make electric charging more economically viable for a diverse range of users. This approach aligns with principles of fairness, ensuring that the benefits of electric mobility are accessible to individuals across different income brackets.
The adoption of AI-driven solutions in electric vehicle (EV) charging is rapidly advancing, offering potential benefits in efficiency, user experience, and grid management.
However, this technological advancement also raises important considerations around algorithmic fairness. Ensuring that AI systems in EV charging are fair and unbiased is critical to promoting equitable access to charging infrastructure.
Diverse and Representative Data
To mitigate biases, it’s crucial to ensure that training data is diverse and representative of the entire user base. This involves collecting data from a broad range of geographic locations, demographic groups, and charging scenarios. Within each dataset biases present in the training data need to be identified and rectified. Below are the various aspects that need to be considered when picking the datasets:
- Geographic Diversity:
- Urban and Rural Areas: Incorporating data from both urban and rural environments ensures that charging grid designs are inclusive and cater to the needs of diverse communities.
- Different Climates: Climate variations impact charging behaviors and energy consumption. Datasets reflecting diverse climate conditions contribute to robust AI models.
- Demographic Diversity:
- Socioeconomic Factors: Including data from various socioeconomic backgrounds helps avoid biases and ensures that charging infrastructure is accessible to users across different income levels.
- Cultural Considerations: Cultural preferences and lifestyle differences influence charging habits. Diverse datasets encompassing cultural nuances contribute to more inclusive charging grid designs.
- Vehicle Diversity:
- Various EV Models: Different electric vehicle models have distinct charging requirements. Incorporating data from a variety of EVs ensures that the charging infrastructure caters to the specifications of various vehicles.
- Charging Technologies: Datasets should account for different charging technologies, including fast charging, standard charging, and emerging technologies, to optimize grid designs accordingly.
- Temporal Diversity:
- Seasonal Variations: Charging behaviors can vary seasonally. Datasets covering different seasons enable AI systems to adapt charging grid designs to changing weather conditions.
- Time-of-Day Patterns: Understanding variations in charging demand throughout the day aids in optimizing charging infrastructure for different timeframes.
While building an AI model for demand prediction- let’s say predicting where to place the next EV charging station, it is crucial to ensure a diverse dataset including all the above features is curated.
Once the features are curated, is it important to access the balance of the dataset. An imbalanced dataset can lead to skewed and biased results. The graphs show balanced data for some of the pivoted features such as Age and Vehicle type preference.
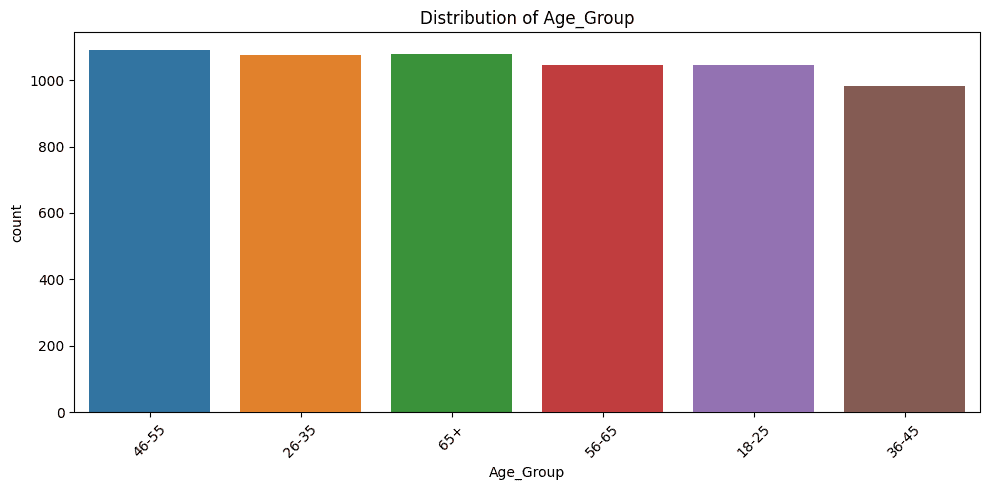
Figure 5: Balanced features for EV charging station placement model by age

Figure 6: Balanced features for EV charging station placement model by vehicle type
Algorithmic Transparency
Transparency is a cornerstone of addressing bias in AI. Charging algorithms should be designed to be transparent, providing users with insights into how decisions are made regarding charging rates, optimal times, and other critical factors. Understanding the algorithm’s decision-making process fosters trust and allows users to hold charging providers accountable.
LIME (Local Interpretable Model-agnostic Explanations) plays a crucial role in enhancing the explainability of AI predictions. By creating interpretable models that approximate the predictions of complex machine learning models, LIME provides insights into how different features influence these predictions. For instance, in the context of EV charging station placement, LIME can help reveal the reasons behind a model’s recommendation to place a charging station- in the below explainer plot- the features that contribute positively to the prediction(placing an EV charging station at location x) is highly impacted by the socio-economic status. Traffic and population density negatively impact the prediction. This is just a hypothetical dataset and analysis, and real life predictions could deeply vary. The purpose of this plot is to show how powerful LIME can be to explain how a particular prediction is made- what features carry more importance vs the others.

Figure 7: Explainable AI for a EV Charging Station prediction using LIME
The EVI-Equity: Electric Vehicle Infrastructure for Equity Model developed by NREL [5] is a fantastic tool for measuring the equity of the nationwide electric vehicle (EV) charging infrastructure using comprehensive, high-resolution analysis. It provides a visualization map to allow stakeholders to examine equity characteristics of EV charging infrastructure making it easy to inspect and understand the results. For eg. when applied to the greater Chicago region, the graph below illustrates the disparate charging access and associated EV adoption based on income and race.

Figure 8: EVI-Equity model results for the greater Chicago region
Protecting User Privacy
With the rapid rise of Connected Vehicles, there is an increasing amount of data being streamed from vehicles to the cloud. This not only includes vehicle metrics such as battery capacity, remaining range, user settings such as climate control, but also driver behavior metrics such as rate of acceleration/braking, video and audio feeds, anti-braking/lane departure sensor activation. These metrics, if used unfairly, can be used to create a behavioral profile for the driver and in-turn add bias into decision making.
As AI processes this vast amount of user data to optimize charging grid placement, privacy becomes a paramount concern. Implementing privacy-by-design principles ensures that AI-driven charging infrastructure respects user privacy and complies with data protection regulations.
Privacy Techniques for Responsible Data Handling:
- Anonymization: Anonymization involves the removal or encryption of personally identifiable information from the data stream. By dissociating the data from specific individuals, it becomes significantly harder to trace metrics back to a particular driver.
- Aggregation: Aggregation involves combining multiple data points to form generalized summaries. Instead of processing individual driver behavior metrics, AI can analyze aggregated patterns across a larger dataset. This not only safeguards the privacy of individual drivers but also ensures that charging grid decisions are based on collective trends rather than specific user profiles.
- Differential Privacy: Differential privacy adds noise or randomness to individual data points, making it challenging to determine the contribution of a single user to the dataset. This technique strikes a balance between data utility and privacy protection, enabling AI to generate accurate charging grid optimizations without compromising the individual privacy of drivers.
- Homomorphic Encryption: Homomorphic encryption enables computations on encrypted data without decrypting it. This technique allows AI to analyze encrypted driver behavior metrics, ensuring that the privacy of individual users is maintained throughout the optimization process. It’s a powerful tool for striking a balance between data-driven insights and privacy protection.
As the global adoption of electric vehicles (EVs) gains momentum, charging networks infused with AI face both promising opportunities and significant responsibilities. Their mission involves providing drivers with convenience and reliability while ensuring the resilience of local grids, all while prioritizing equity and accountability. Although the challenges are intricate, the potential future benefits are immense, ranging from cleaner air and climate change mitigation to achieving energy independence and fostering the development of next-generation skills.
The pivotal role of AI and machine learning in bringing this vision to fruition cannot be overstated. These technologies hold the promise of orchestrating serialized, personalized charging on a massive scale, catering to millions of users. However, to secure public trust, the algorithms driving these systems must center on principles of fairness and transparency, all while enhancing accessibility and reliability.
[1] US electric vehicle charging market growth: PwC
[2] The role of artificial intelligence in the mass adoption of electric vehicles
[3] Data-driven smart charging for heterogeneous electric vehicle fleets – ScienceDirect
Swagata Ashwani is a seasoned data scientist with a rich background in analytics and big data. Currently serving as the Principal Data Scientist at Boomi, Swagata plays a crucial role in harnessing the power of data to drive innovation and efficiency. In her role, she plays a crucial role in leading generative AI initiatives for the company. She is also Chapter Lead at SF Women in Data, where she fosters building a rich community for women to celebrate women in varied data roles.
Ankur Gupta is an engineering leader with a decade of experience spanning sustainability, transportation, telecommunication and infrastructure domains; currently holds the position of Engineering Manager at Uber. In this role, he plays a pivotal role in driving the advancement of Uber’s Vehicles Platform, leading the charge towards a zero-emissions future through the integration of cutting-edge electric and connected vehicles.