The Impact of Data Analytics Integration Mismatch on Business Technology Advancements
Data analytics has become a cornerstone of calculated decision-making. Unfortunately, integration mismatches are common — and can do more harm than good. If organizations try to solve the problem with cleaning alone, their technology advancement strategies will suffer. Will integration errors permanently affect their business outcomes?
How Does a Data Analytics Integration Mismatch Happen?
Data analytics integration mismatches happen when businesses combine multiple sources or systems improperly. The resulting discrepancies — missing, inaccurate, incomplete or duplicate information — force them to postpone their analysis until after cleaning.
These integration failures happen for several reasons. Sometimes, it’s because businesses use legacy systems or software that are incompatible with their newer technology. For example, one might be NoSQL while the other is hierarchical — these databases have different schemas and queries that are expensive and time-consuming to fix.
Other times, integration errors happen because data sources are formatted differently. For example, one system might use JSON while the other uses XML. Alternatively, the content can be inconsistent. For instance, while a download and an install sound like the same thing, they represent different actions — categorizing them as the same can lead to discrepancies.
Although resolving integration mismatches may seem simple, it can be challenging in practice. That might be why only 13% of business executives feel their organization’s data strategy is paying off. Realistically, an incomplete or substandard analysis leads to poor insights.
The Impact of Bad Integrations on Business Advancement
Data analytics integration mismatches can interrupt — or even stop — business technology advancement.
- Higher Operational Expenses – Cleaning and transforming information to mitigate mismatches is time-consuming. If you’re like many professionals, you might waste up to 40% of your workweek on data management. The more time you spend fixing integration errors, the less money you’ll have left over to fund innovative technological advancements.
- Poor Business Outcomes – You might not always realize you have analytics integration mismatches — meaning you may believe the data-driven insights you receive are accurate. In reality, the system and information discrepancies create a faulty analysis. When you act on it, you invest in irrelevant technology advancements. In other words, your business outcomes worsen.
- Delayed Decision-Making – If you experience analytics integration errors, you’re forced to prioritize scrubbing duplicate and incomplete information. According to some estimates, data analysts spend 80% of their time on cleaning and only 20% on analysis. You likely have to delay decision-making as a result, meaning you lose out on precious strategizing time.
- Competitive Disadvantages – Tracking web traffic, sales and conversions is supposed to give you a competitive advantage. You can’t optimize services, improve marketing efforts or better understand customer behavior when you have integration mismatches. When faulty data leads to incomplete insights, the only result is a competitive disadvantage.
- Missed Business Opportunities – Even if you know about your data analytics integration errors, you still may be unable to secure the technology advancements you crave. Frankly, you might not know how to proceed without insight from an analysis. Even if that’s not the case, your mismatches still halt innovation. What’s the point of adopting emerging technology for incompatible systems?
Ways to Prevent Data Analytics Integration Mismatches
Data analytics can be strategically beneficial when done right. According to one survey, 43% of organizations reported it gave them a competitive advantage. Another 56% of respondents claimed it enhanced their decision-making process. Needless to say, businesses should prioritize preventative efforts.
While data cleaning and preprocessing can fix low-quality data after collection and aggregation, businesses’ efforts are better spent mitigating the issue altogether. Proactive action can prevent analytics integration mismatches, enabling them to divert their time and funds toward emerging technological advancements.
A strategic data collection process ensures little to no inaccurate or incomplete entries enter the aggregation phase. This way, analysts spend less time on inefficient cleaning and transformation practices — and businesses can get their insights faster.
Uniformity is crucial. Businesses should standardize their data formats and structures to minimize the bad information they process. For example, when tracking customers’ full names, they should ensure the systems always put the individual’s first name before the last name or vice versa to prevent duplicates and missing values.
While regulations and budget constraints often force businesses to hold onto outdated technology, phasing them out is beneficial. Businesses should consider restructuring to ensure their systems and software are compatible — even if it means accepting a temporary downgrade. The sacrifice will pay off if it enables them to innovate in the future.
Proactivity Prevents Data Analytics Integration Mismatches
When professionals are aware their data sets have missing, incomplete or inaccurate values, cleaning is often their first instinct. While it is an adequate approach, it won’t result in the same benefits as proactive action. If businesses prioritize standardization and compatibility, they can gain a competitive advantage, improve their strategic decision-making and accelerate technological advancement.
About the Author
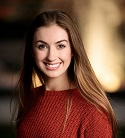
April Miller is a senior IT and cybersecurity writer for ReHack Magazine who specializes in AI, big data, and machine learning while writing on topics across the technology realm. You can find her work on ReHack.com and by following ReHack’s Twitter page.
Sign up for the free insideBIGDATA newsletter.